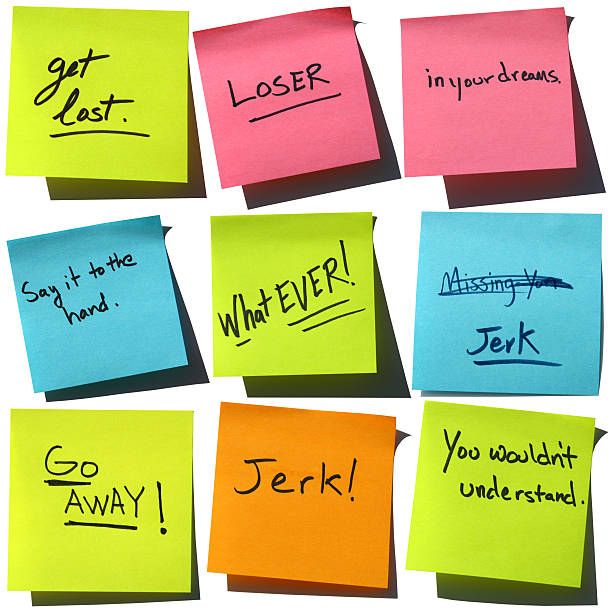
My Conversations with ChatGPT
Machine Translation (MT) and translations generated by AI have brought about a paradigm shift in the translation industry. The output of MT will continue to improve, especially when associated with clients’ translation memories and human editing. Language Service Providers are adapting and are including MT in their processes, when MT is applicable. While linguists will continue to be essential in the process, their role will shift from that of translators to validators of content generated by MT. At Excel Translations, we wanted to know what AI thinks about all of this and decided to have an open conversation on the matter with ChatGPT. I will regularly publish a question and ChatGPT’s response, uncensored.
QUESTION 42:
How can machine translation models be made aware of the ongoing evolution of languages, including emerging slang, shifts in meanings, and grammatical changes?
Chat GPT’s Response:
Machine translation models can be made aware of the ongoing evolution of languages through a combination of techniques:
- Continuous Learning: Implement mechanisms for models to continuously learn from up-to-date and diverse language data sources, such as social media, forums, and news articles, to stay informed about new terms, slang, and shifts in language use.
- Dynamic Vocabulary Expansion: Develop strategies to dynamically update the model’s vocabulary with emerging words, idioms, and expressions, ensuring it remains current and aligned with evolving language trends.
- Adaptive Training: Regularly fine-tune the model on recent and relevant data to capture the latest linguistic variations, allowing it to adapt to shifts in meanings and grammatical changes.
- Slang and Contextual Knowledge: Incorporate specialized training data that focuses on slang, cultural references, and context-dependent language use to enhance the model’s understanding of colloquialisms and nuances.
- Multi-Source Data: Combine diverse data sources, including multimedia content like images, videos, and audio, to provide a richer context and a broader view of language evolution.
- Human Feedback: Encourage user feedback and corrections to help the model learn from real-world language evolution instances and refine its translations over time.
- Active Learning: Develop strategies to actively select and prioritize data for model training that reflects emerging language patterns, thereby improving the model’s responsiveness to changes.
- Collaborative Platforms: Engage linguistic experts, native speakers, and language enthusiasts to contribute to curated datasets that showcase evolving language dynamics, including slang and shifts in meanings.
By leveraging these techniques, machine translation models can be equipped to better understand and adapt to the ongoing evolution of languages, capturing emerging slang, shifts in meanings, and grammatical changes to provide more accurate and contextually relevant translations.
Leave a Reply