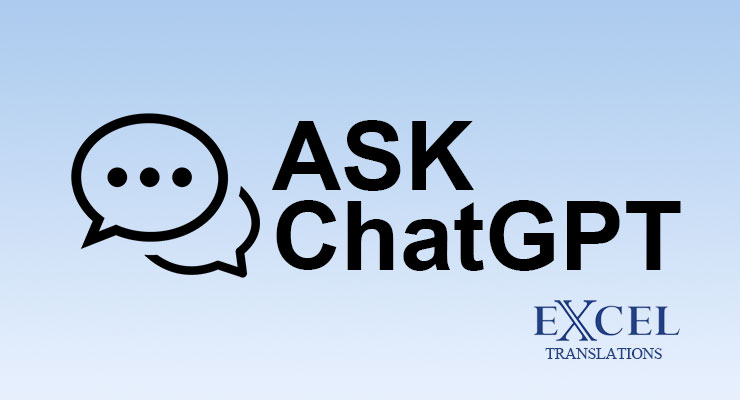
My Conversations with ChatGPT
Machine Translation (MT) and translations generated by AI have brought about a paradigm shift in the translation industry. The output of MT will continue to improve, especially when associated with clients’ translation memories and human editing. Language Service Providers are adapting and are including MT in their processes, when MT is applicable. While linguists will continue to be essential in the process, their role will shift from that of translators to validators of content generated by MT. At Excel Translations, we wanted to know what AI thinks about all of this and decided to have an open conversation on the matter with ChatGPT. I will regularly publish a question and ChatGPT’s response, uncensored.
QUESTION 45:
How can machine translation be improved for languages with limited training data, also known as low-resource languages?
Chat GPT’s Response:
Improving machine translation for low-resource languages involves creative strategies:
- Transfer Learning: Fine-tune pre-trained models on the target language to leverage shared features from high-resource languages.
- Data Augmentation: Generate synthetic parallel data by translating monolingual text in both directions.
- Multilingual Training: Train models on multiple low-resource languages together to benefit from shared characteristics.
- Unsupervised Methods: Develop models that learn to translate using only monolingual data without parallel pairs.
- Domain Focus: Collect and use domain-specific data to enhance translation quality in specific contexts.
- Human Collaboration: Combine machine translation with human translators for complex language elements.
- Linguistic Insight: Integrate language-specific rules and structures to guide translation.
These approaches collectively address the challenge of limited training data, enhancing translation capabilities for low-resource languages.
Leave a Reply