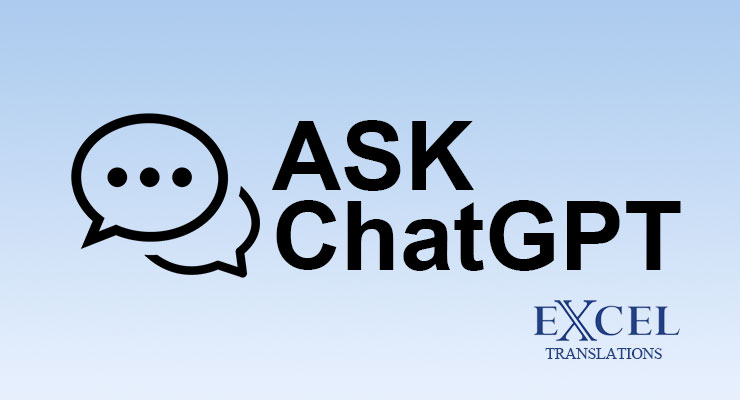
My Conversations with ChatGPT
Machine Translation (MT) and translations generated by AI have brought about a paradigm shift in the translation industry. The output of MT will continue to improve, especially when associated with clients’ translation memories and human editing. Language Service Providers are adapting and are including MT in their processes, when MT is applicable. While linguists will continue to be essential in the process, their role will shift from that of translators to validators of content generated by MT. At Excel Translations, we wanted to know what AI thinks about all of this and decided to have an open conversation on the matter with ChatGPT. I will regularly publish a question and ChatGPT’s response, uncensored.
QUESTION 64:
What are some of the top challenges in creating machine translation software?
Chat GPT’s Response:
Some of the top challenges in creating machine translation software include:
- Data Availability: Obtaining high-quality parallel text data for training models, especially for less common languages, can be a significant challenge.
- Model Complexity: Designing and training complex neural network architectures, such as transformers, requires expertise and substantial computational resources.
- Domain-Specific Translation: Adapting machine translation to specific domains like legal, medical, or technical texts can be difficult due to specialized terminology.
- Maintaining Quality: Ensuring translation quality and preventing the propagation of biases in translations is an ongoing challenge.
- Resource Requirements: Machine translation projects require significant computational resources, both for training and deployment.
- Evaluation Metrics: Developing reliable metrics to measure translation quality accurately is a challenge.
- Ethical Concerns: Addressing ethical issues related to bias, privacy, and cultural sensitivity is crucial.
- Post-Editing: In many cases, human post-editing is required to improve translation quality, which can be time-consuming and costly.
- Scaling to Multiple Languages: Expanding translation capabilities to cover a wide range of languages adds complexity and data requirements.
- Real-time Translation: Achieving real-time or low-latency translation for applications like chatbots or video conferencing can be challenging.
- Resource-Scarce Languages: Developing accurate translations for languages with limited linguistic resources is particularly challenging.
These challenges require a combination of linguistic expertise, machine learning knowledge, access to data, and computational resources to create effective machine translation software.
Leave a Reply