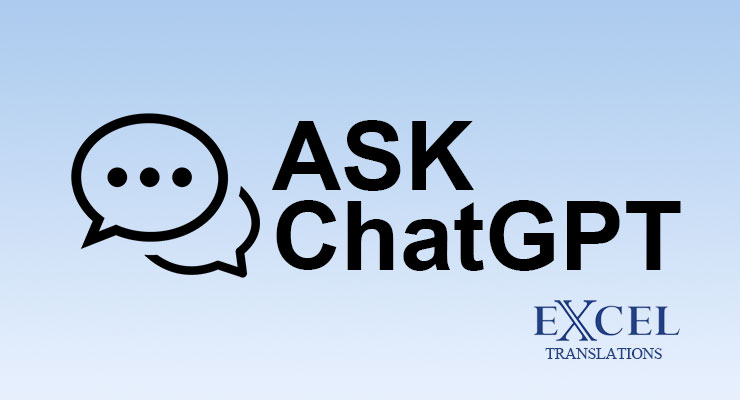
My Conversations with ChatGPT
Machine Translation (MT) and translations generated by AI have brought about a paradigm shift in the translation industry. The output of MT will continue to improve, especially when associated with clients’ translation memories and human editing. Language Service Providers are adapting and are including MT in their processes, when MT is applicable. While linguists will continue to be essential in the process, their role will shift from that of translators to validators of content generated by MT. At Excel Translations, we wanted to know what AI thinks about all of this and decided to have an open conversation on the matter with ChatGPT. I will regularly publish a question and ChatGPT’s response, uncensored.
QUESTION 138:
Is it easy for LSP’s to train their MT engine?
Chat GPT’s Response:
Training a machine translation (MT) engine can be a complex and resource-intensive process, but it’s not necessarily easy. Language Service Providers (LSPs) often have the expertise and resources to undertake MT training, but several factors influence the ease or difficulty of the process:
Data Availability: The availability of high-quality, domain-specific training data is crucial for training an effective MT engine. LSPs may have access to large corpora of translated texts, which can be used to train the engine. However, curating and cleaning this data to ensure accuracy and relevance can be time-consuming.
Expertise: Training an MT engine requires expertise in natural language processing (NLP), machine learning, and linguistics. LSPs with in-house expertise in these areas may find it easier to train and fine-tune MT models compared to those without specialized knowledge.
Infrastructure: Training MT models requires significant computational resources, including high-performance servers and specialized hardware such as GPUs. LSPs need to invest in infrastructure or cloud computing services capable of handling the computational demands of training large-scale MT models.
Evaluation and Iteration: Training an MT engine is an iterative process that involves training, evaluation, and fine-tuning based on performance metrics and user feedback. LSPs need to establish robust evaluation frameworks and workflows to assess the quality of the MT output and identify areas for improvement.
Adaptation to Client Needs: LSPs often serve clients with diverse linguistic and domain-specific requirements. Training an MT engine that accurately translates content across different languages and domains requires customization and adaptation to meet the specific needs of each client.
Integration with Workflows: Integrating the MT engine into existing translation workflows and tools is essential for seamless adoption and usability. LSPs need to ensure compatibility with translation management systems (TMS), CAT tools, and other software used in their operations.
While training an MT engine presents challenges, LSPs with the necessary expertise, resources, and commitment to quality can successfully develop and deploy effective MT solutions to enhance their translation services. Additionally, leveraging pre-trained MT models or collaborating with MT providers can offer alternatives for LSPs looking to incorporate MT capabilities into their offerings without undertaking full-scale training efforts from scratch.
Leave a Reply