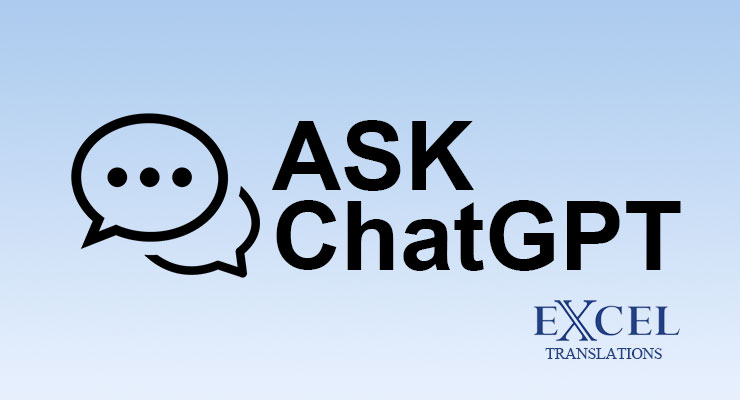
My Conversations with ChatGPT
Machine Translation (MT) and translations generated by AI have brought about a paradigm shift in the translation industry. The output of MT will continue to improve, especially when associated with clients’ translation memories and human editing. Language Service Providers are adapting and are including MT in their processes, when MT is applicable. While linguists will continue to be essential in the process, their role will shift from that of translators to validators of content generated by MT. At Excel Translations, we wanted to know what AI thinks about all of this and decided to have an open conversation on the matter with ChatGPT. I will regularly publish a question and ChatGPT’s response, uncensored.
QUESTION 41:
What are the recent advancements in machine translation research?
Chat GPT’s Response:
As of my last knowledge update in September 2021, there have been several exciting advancements in machine translation research. Please note that these advancements might have evolved further since then. Here are some notable recent trends and developments:
- Transformer Models: Transformer-based architectures, such as the “Transformer” model and its variants (like BERT, GPT, T5), have revolutionized machine translation. These models incorporate self-attention mechanisms to capture contextual information efficiently and have led to significant improvements in translation quality.
- Pre-trained Language Models: Large pre-trained language models like BERT and GPT-3 have been fine-tuned for machine translation tasks. This transfer learning approach has shown promise in improving translation quality, especially for low-resource languages.
- Multilingual and Zero/Few-Shot Translation: Researchers have been working on models that can perform translation between multiple languages, even if they were not seen during training. Zero-shot and few-shot translation aims to translate between language pairs with minimal or no direct training data.
- Neural Architecture Search: Researchers are using neural architecture search techniques to automatically discover more effective neural network architectures for machine translation, leading to more efficient and accurate models.
- Domain Adaptation: Models that can adapt to specific domains, such as medical, legal, or technical translation, have been explored. This involves fine-tuning models on domain-specific data to improve translation accuracy in specialized fields.
- Quality Estimation and Post-Editing: Advances in quality estimation models help predict the quality of machine-generated translations, aiding human post-editors in the translation process.
- Multimodal Translation: Research has been focused on translating not only text but also other modalities like images and speech. This involves combining vision and language models for more accurate and contextually rich translations.
- Attention Mechanisms: Continued research into attention mechanisms has led to improvements in how models focus on relevant parts of the input text, leading to better translation quality.
- Low-Resource Languages: Efforts to improve translation for languages with limited training data have gained attention. Techniques like transfer learning, data augmentation, and leveraging related languages have been explored.
- End-to-End Models: Researchers are working on end-to-end translation models that can directly translate spoken language to a target language, eliminating the need for intermediate text representation.
- Post-Editing and Human-in-the-Loop Systems: Combining machine translation with human involvement, such as interactive post-editing, to create more accurate and contextually appropriate translations.
- Ethical Considerations and Bias: As machine translation becomes more widespread, there’s a growing focus on addressing biases in translations and ensuring fairness and cultural sensitivity.
Keep in mind that the field of machine translation is rapidly evolving, and new advancements are likely to have emerged since my last update. To get the most up-to-date information, I recommend checking recent research papers, conferences, and resources in the field.
Leave a Reply