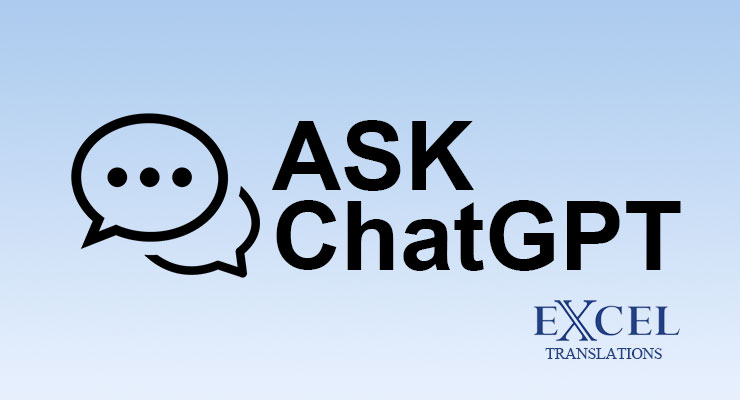
My Conversations with ChatGPT
Machine Translation (MT) and translations generated by AI have brought about a paradigm shift in the translation industry. The output of MT will continue to improve, especially when associated with clients’ translation memories and human editing. Language Service Providers are adapting and are including MT in their processes, when MT is applicable. While linguists will continue to be essential in the process, their role will shift from that of translators to validators of content generated by MT. At Excel Translations, we wanted to know what AI thinks about all of this and decided to have an open conversation on the matter with ChatGPT. I will regularly publish a question and ChatGPT’s response, uncensored.
QUESTION 46:
How can machine translation systems be adapted to specific domains (e.g., medical, legal, technical) to improve translation quality?
Chat GPT’s Response:
Machine translation systems can be adapted to specific domains by employing domain-specific training data and fine-tuning techniques. Here’s how:
- Domain-Specific Data: Gather a substantial amount of domain-specific bilingual data, such as medical, legal, or technical texts, that reflect the terminology, style, and context of the target domain.
- Fine-Tuning: Pretrain a general-purpose machine translation model on a large dataset and then fine-tune it using the domain-specific data. This fine-tuning process helps the model learn the nuances and terminology specific to the domain.
- Vocabulary Control: Incorporate domain-specific glossaries, terminology lists, and vocabulary constraints during training and decoding to ensure accurate translation of specialized terms.
- Adaptive Training: Implement techniques that assign more weight to domain-specific data during training, allowing the model to better capture domain-specific language patterns.
- Transfer Learning: Leverage transfer learning by initializing the model with a pretrained general model and then adapting it to the domain, minimizing the need for extensive training from scratch.
- Evaluation and Iteration: Continuously evaluate the performance of the adapted system using domain experts and gather feedback for further refinement.
- Post-Editing Collaboration: Involve domain experts to perform post-editing of machine-generated translations, providing feedback that can be used to further improve the system’s domain-specific capabilities.
- Use Case Customization: Customize the system’s behavior based on the specific requirements of the domain. For instance, medical translations might prioritize accuracy over fluency, while marketing translations could emphasize creative language use.
By employing these strategies, machine translation systems can be adapted to specific domains, leading to improved translation quality that aligns with the specialized terminology and context of the target field.
Leave a Reply